Groundspeed was acquired by Insurance Quantified in 2023.
In today’s data-driven world, the accuracy and reliability of data are crucial for organizations across various industries. As an insurance carrier, you rely on data to make informed underwriting decisions, and you face the challenge of balancing data quality, speed, and cost. You know you need to be the fastest to quote, but you also want to ensure that you write profitable business for your carrier.
At Groundspeed, we created our AI pipeline to transform the commercial underwriting process by providing near-perfect risk data in just hours, so that underwriters can quickly quote and win profitable new business.
In this blog post, we will explain how Groundspeed provides high-quality risk data with our Quality Assurance Process, which is the best in the industry and delivers over 98% data point accuracy without sacrificing speed.
Data You Can Trust
Groundspeed monitors the quality of the data we send our customers at various points in our AI pipeline. We ensure the quality of each data point delivered to customers using automated in-production quality controls. Audits by internal subject matter experts enable continuous improvement of our data pipeline over time. That way, carriers get accurate data for the fields that are critical to their success, such as:
- Line of Business
- Subcoverage
- Net Total Incurred and other financials
- Policy Year
At Groundspeed, we think about quality in terms of accuracy at the data point level. We do that to ensure that carriers receive consistent quality across all documents that Groundspeed processes. A “data point” means a specific value for a particular field. For example, one claim could have a Net Total Incurred value of $10,000, while another claim could have a value of $20,000. Those two values for Net Total Incurred would be two different data points.
Let’s learn more about the “why” and the “what” of data quality at Groundspeed.
Five Data Quality Goals
When our team created our Quality Assurance model, we set a few goals to ensure we provide our customers with the highest quality data.
- Consistent: We strive to keep our data quality consistent across all customers and all data products.
- Measurable: We aim for quantified measurement of all data points, including raw capture and computed field categories.
- Traceable: We strive to make measurements traceable at the level of each operation on a data point to enable ongoing product improvements.
- Transparent: We strive to provide consistent transparency to customers and the Groundspeed team in order to manage root-cause issues and gain end-user confidence.
- Continuous Improvement: We aim to integrate continuous feedback and improvement loops into all our automated and human-in-the-loop tooling to maintain consistency in quality as production conditions change over time.
Now that you know our goals for our Quality Assurance model, let’s take a closer look at how we perform our data checks and audits.
How does Groundspeed Measure Data Accuracy?
The Groundspeed Quality Assurance environment ensures that our AI models, which enable automated extraction and normalization, and Human in the Loop system that validates data in production, are in control and yield data point accuracy above 98%.
Groundspeed monitors quality at the data-point level through quality controls and regular audits. This multi-faceted approach uses two sets of automated QA checks called “Automation Validation” and “In-Line Quality Assurance,” in addition to several audit protocols. When put together, these enable us to monitor and detect anomalies in our models and ensure that all systems function correctly.
The First Line of Defense: Quality Checks
Every file that Groundspeed processes is automatically checked before its data is delivered to customers. These checks determine whether a data point is likely to be correct based on the captured value and field type.
Automation Validation
Document processing automation is critical to Groundspeed’s AI with Human in the Loop system. Every time an automation runs, we check that expected data values were captured and that the values produced match what we expect to see in their field. When there’s a likely mismatch, we send the file to a human for review. For example, we expect every non-summary claim record to have an alphanumeric claim number associated with it.
(For more, read Delivering on the Promise of Automation in Commercial Insurance.)
In-Line Quality Assurance Checks
In cases when an automation is unable to fully process a file, a user finishes capturing data from the file. Once that capture is done, we use In-Line Quality Assurance to check the file for data accuracy. This ever-evolving system is the product of years of work by Groundspeed subject matter experts, tempered by customer feedback, and ensures high quality across the full range of insurance documents processed by Groundspeed.
Example: In-Line Quality Assurance
Let’s look at an In-Line Quality Assurance example of a capture error. On this ACORD Form, our system found an OCR error before delivery that would have otherwise been put into these fields in our data output. The system flagged that the original captured effective date had unexpected content, and a user was directed to correct this before this data was returned to our customer.
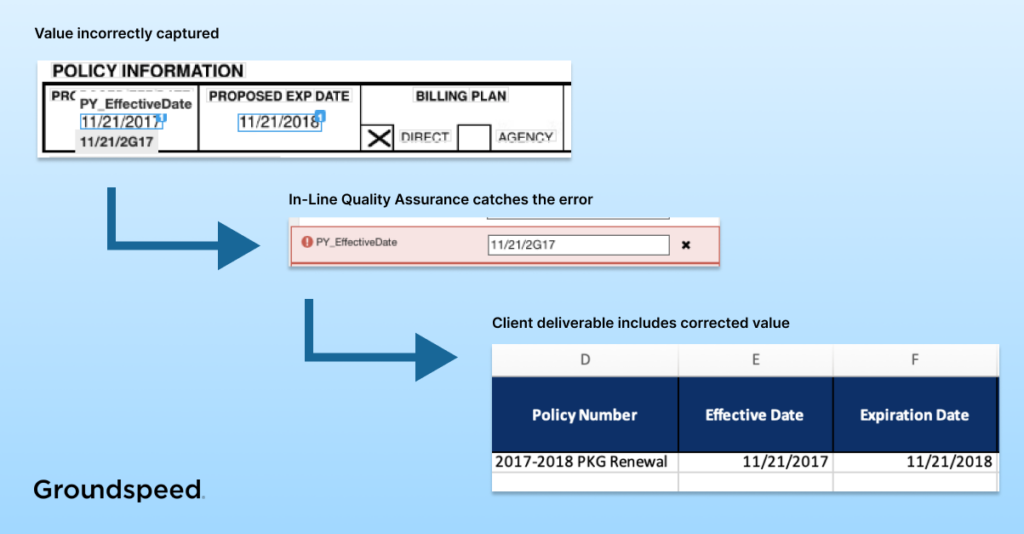
The Second Layer of Data Quality Assurance: Auditing
Every day our Audit Team checks the accuracy of data points in a sample of AI predictions and Human in the Loop additions and makes corrections when necessary. These corrections provide training data inputs for automation improvements and help us fine-tune our human review standard operating procedures.
(To learn more about how Groundspeed uses human review to ensure high data coverage, accuracy, and speed at a low cost, read our blog post, The End of Underwriting Tradeoffs.)
Post-Production Audits
By auditing a statistically significant sample size of previously delivered production files (try saying that three times fast!), our Audit Team monitors data quality in order to guide root cause analysis and continuous improvements in our models.
Machine Learning Model Auditing
Auditors also regularly examine the predictions from Groundspeed’s Machine Learning models to ensure that the models continue delivering high-quality outputs. We use these findings to make adjustments that keep our models returning nearly perfect data point quality, even as the data that Groundspeed receives changes over time.
Access High-Quality Data with Groundspeed’s Underwriting AI
Providing high-quality data is core to our mission at Groundspeed. On a daily basis, we strive to ensure that we give our customers near-perfect data so that they can quote more business profitably. If you want to transform your underwriting process by accessing high-quality risk data, schedule a call with a Groundspeed team member today. We would be delighted to show you why Groundspeed’s underwriting AI is the best in the industry.